Data quality is a critical aspect of any effective AML programme. In the ever-evolving landscape of financial crime, ensuring the accuracy, completeness, and reliability of data is paramount for successfully combating money laundering. However, the state of play in relation to AML data is far from perfect. Many organisations still struggle with data issues, hindering their ability to detect and prevent illicit financial activities. To overcome these challenges it is essential to understand the red flags, how to implement measures to mitigate them, and what best practices your organisation can continually return to in order to enhance data quality and strengthen your overall AML efforts.
WHAT DOES THE RESEARCH TELL US?
- Poor data leads to increased regulatory scrutiny and reputational damage
FATF notes that poor data quality can lead to missed suspicious activity, which can contribute to money laundering and terrorist financing. - Increase in fines
US$36 billion in AML fines since 2008 (~50% of those fines disturbed in the past 4 years). - Data quality issues are 40% of all IT workload
In a study by Gartner, data quality issues account for 40% of all IT workload. Poor data quality requires additional resources to clean, validate, and integrate data, which can impact the efficiency of an AML programme. - Heavy cost of false positives
A study by Deloitte shows nearly 60% of respondents indicated that they were struggling with a significant number of false positives and the growing complexity of the task. The study indicates the value of sufficiently tuning rule typologies and thresholds can significantly reduce the cost of false positives.
“Survey respondents identified key challenges in the areas of technology, process, and people. The problems range from technology-related issues (lack of data accuracy and sufficiency, and increased false positives), and process related issues (limited coverage of known TM red flags) to people-related issues (dependencies on manual processes). Similarly, the issues related to the lower confidence level in screening solution (besides data accuracy and sufficiency, and false positives) include inadequate fuzzy logic capabilities and list management. Fines imposed recently on banks indicate that these issues are a part of ongoing struggle of banks, and question their efficacy of meeting AML compliance and regulatory expectations.”
AML DATA RED FLAGS AND CONTRIBUTING FACTORS
Do you have the protocols and procedures in place that would let you know if red flags were occurring in your organisation? Consider the controls and tools you have in place and the idea of “you don’t know, what you don’t know”.
- High error rates – If the data within your AML programme contains a high number of errors or inconsistencies, this may indicate poor data quality. The errors could be in the data entry or processing stages, or result from poor data management practices.
- Incomplete data – Do you know the limitations of your core systems? If controls are missing you should speak to your suppliers. If there is incomplete or missing data, it may be difficult to fully assess AML risk.
- Data inconsistencies – If there are inconsistencies in the data, this may indicate poor data quality. Inconsistencies could arise when data is collected from different sources, or if there are errors in data processing.
- Low data accuracy – If the data is inaccurate, it may be challenging to draw reliable conclusions from the data analysis.
- Data duplication – Duplicate data can cause confusion and lead to inaccurate conclusions.
- Poor data integration – If data is not integrated correctly, it may be challenging to access the data when it is needed. This can lead to data silos, where different departments or systems hold different data that is not integrated.
- Poor data protection – Data protection, against internal bad actors is paramount, particularly in a post-covid world where staff can perform their jobs from home the appropriate environmental controls can be lacking.
- Poor data governance – Poor data governance practices can result in poor data quality. This can be seen if there are no clear guidelines for data management, or if there are inadequate controls to ensure data quality.
HOW TO GET THE BASICS RIGHT
First and foremost, adopt best practices, here are the five I keep returning to:
- Standardise – You can easily integrate and analyse
- Test and validate
- Automate – Remove the element of human error wherever possible
- Govern – You should at least have “AML data” as a discussion item in your regular internal and supplier meetings
- Analyse – Regular analysis of your AML data will prevent your gold standard from slipping
MITIGATING THE RED FLAGS
- Champion compliance culture
You have to have staff that want to mitigate and challenge the “it is what it is” mentality, collaborate across teams and prevent data quality issues before they occur. The key word here is “champion”. What are you doing to uphold the appropriate compliance culture so it successfully filters down to all reporting lines? - Data training
You cannot solve these issues if you do not understand the data ecosystem you’re operating in. Any staff member that is working in a decision making capacity should fully understand the data they are using to make those decisions and the genesis of that data. - Leverage AML software
Remove the risk of human error. What controls does your core system have? Can your supplier introduce additional controls? As a customer, your input should be valued and taken into consideration, as it ultimately contributes to the improvement and marketability of a product. - Leverage your rules / Data integrity rules
A good Transaction Monitoring system should allow you to create rules that cater to your specific needs, including data health. You should be able to go behind the scope of AML Transaction Monitoring rules and create alerts for missing properties, inaccurate data and incomplete data.
AML DATA GOLD STANDARDS
So what does good data look like?
- Accurate: Free of errors, inconsistencies, and duplicates. It is reliable and can be trusted to provide an accurate view of customers and transactions.
- Complete: Includes all necessary data points to provide a comprehensive view of customers and transactions. This can include personal information, transaction history, and risk assessments.
- Consistent: Follows a standardised format and is consistent across all systems and processes. This ensures that data is easily searchable and can be easily integrated and analysed.
- Timely: Updated in real-time or near-real-time, ensuring that financial institutions have access to the most up-to-date information when making decisions.
- Relevant: Relevant to the specific needs of the AML programme. This can include data that is specific to a particular customer segment, geography, or product line.
- Single source of truth: Comprehensive view of customers and transactions.
- Secure: Particularly from a standpoint of internal bad factors. Are your staff working from home? What environmental controls are in place?
HOW TO SELL THE GOLD STANDARD
KNOW THE RESEARCH AND REGULATORS POSITION
Understand your data ecosystem, regulatory and humanitarian risks and your limitations.
UNDERSTAND RED FLAGS AND HOW TO MITIGATE
Get the basics right, champion compliance culture and leverage your AML software.
BUILD YOUR CASE AND SELL THE GOLD STANDARD
Know your audience, explain the value and be brave.
At Jade ThirdEye, we understand the nature of automated AML compliance software and hurdles you face. Talk to our team today about your data concerns to learn more.
Get in touch with us!
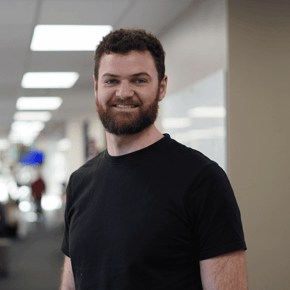